Research conducted by Answers in Genesis staff scientists or sponsored by Answers in Genesis is funded solely by supporters’ donations.
Abstract
Pedigree-based mutation rates act as an independent test of the young-earth creation and evolutionary timescales. Currently, evolutionists use published Y chromosome pedigree-based mutation rates to argue for an ancient origin of humanity. However, their published studies rely on low-coverage sequence runs. We show that pedigree-based mutation rates from high-coverage sequence runs are hidden in the evolutionary literature, and we demonstrate that these rates confirm a 4,500-year history for human paternal ancestry.
Keywords: Y chromosome, molecular clock, mutation rate, pedigree, sequence coverage, phylogenetic tree, young-earth creation, evolution
Introduction
On the timescale of human origins, the young-earth creation (YEC) and evolutionary models contrast by orders of magnitude. According to Scripture, the first humans—Adam and Eve—lived only about 6,000 year ago (Hardy and Carter 2014). Current evolutionary thought puts the origin of modern Homo sapiens upward of 200,000 to 300,000 years ago (e.g., see Karmin et al. 2015). Scientifically, this difference in orders of magnitude should be easily resolvable in multiple scientific fields, especially in the field of human genetics.
Historically, the genetic timescale of human origins has been dominated by circular evolutionary arguments. In theory, to calculate the timescale of human origins, investigators must employ a version of the equation D = RT, where D represents genetic differences, R represents the mutation rate, and T represents the time of origin. However, in decades past, biologists have had data only for D in this equation. Nonetheless, absent an empirical measurement of human mutation rates, evolutionists calculated R by dividing known human genetic diversity into the evolutionary-geology-based time of origin of Homo sapiens—and referred to the result as the “molecular clock.” Obviously, this method derives values for R by first assuming a model-specific value for T—which represents a circular argument, if the goal of the argument is to empirically determine a value for T.
False starts aside, in the last few decades pedigree-based mutation rates have provided an independent test of the YEC and evolutionary timescales—a test free of circular evolutionary assumptions.
Specifically, pedigree-based human mutation rates have directly tested the timescale of human origins in the realm of human mitochondrial DNA (mtDNA) differences. For example, multiple studies over the last two decades have revealed an average mtDNA mutation rate that explains global human mtDNA differences within 6,000 years (Jeanson 2013, 2015, 2016; see also the following exchange: Frello 2017a, 2017b; Jeanson 2017a, 2017b).
In the realm of nuclear DNA differences, pedigree-based human mutation rates have indirectly tested the timescale of human origins. Because YE creationists explain the vast majority of autosomal differences by heterozygosity created in Adam and Eve (Jeanson and Lisle 2016; Sanford et al. 2018), and not via mutations since Creation, a direct molecular clock comparison is not possible for most nuclear DNA differences. However, the YEC model successfully explains the rare autosomal differences by post-Creation mutation (Jeanson and Lisle 2016). Conversely, since evolution explains all autosomal differences by mutation, evolutionists see the rare autosomal differences as stemming from the recent surge in human population growth (Coventry et al. 2010; Fu et al. 2013; Keinan and Clark 2012; Nelson et al. 2012; Tennessen et al. 2012). Consequently, at present, molecular clock analyses of rare human autosomal differences test—and appear to fit—both YEC and evolution.
The one human genetic compartment that has not received as much attention by all sides in the origins debate is the Y chromosome. For YE creationists, their expectations for Y chromosome differences today are easy to derive. Because males are XY and females XX, Adam would have been created XY, and Eve, XX. Therefore, a single Y chromosome would have been present at Creation. Consequently, unless God created Adam’s gametes with Y chromosome differences (Carter, Lee, and Sanford 2018; Sanford et al. 2018), all modern Y chromosome differences would be the result of mutations since mankind’s origin. Conversely, evolutionary expectations are also easy to derive. Because evolutionists explain all genetic differences ultimately by mutation, they also explain all Y chromosome differences by mutation. Thus, in theory, the Y chromosome differences and mutation rates could represent another direct test of the YEC and evolutionary timescales.
To date, two published studies explicitly attempt to obtain the pedigree-based per-generation mutation rate for the Y chromosome (Helgason et al. 2015; Xue et al. 2009). Both studies have reported results to be consistent with the evolutionary timescale.
Taken at face value, these Y chromosome results together with the mtDNA results create a scientific dilemma for both the YEC and evolutionary models. On one hand, the mtDNA pedigree-based mutation rates are consistent with the YEC model. On the other hand, the Y chromosome rates would seem to be consistent with the evolutionary model. Thus, each origins model must explain the contrary data in one genetic compartment, without compromising the supporting data in the other genetic compartment.
A recent observation suggests a path forward. Among evolutionists, it is well-known that low coverage sequencing misses many real Y chromosome differences. For example, Poznik et al. (2016) compared low coverage versus high coverage sequences in 143 individuals. They discovered that missing DNA variants are found throughout the Y chromosome tree. From Supplementary Figure 1 of Poznik et al. 2016, around 36 (i.e., 19 + 17 = 36) Y chromosome variants were missing in nearly all samples examined (i.e., the “140” and “141” columns). Since these variants are found in nearly all samples, they would occupy, by definition, the deep branches on the Y chromosome tree. Conversely, on average, about 23 Y chromosome singleton variants per individual (see the “1” column; 3,343 variants/143 individuals = 23 variants per individual) were missing in low coverage sequences. Again, by definition, singletons are variants at the tips of the Y chromosome tree.
These missing variants at the tips of the tree have the most relevance to our question of pedigree-based mutation rate studies. By definition, comparisons of living fathers and sons represent the most recent time points on the Y chromosome tree—the tips of the tree. Therefore, low coverage studies would likely miss several real Y chromosome (mutational) variants. Since the total number of mutations between fathers and sons is already low/is already a rare event, a discovery of even 10 additional mutations via high coverage sequencing could have a dramatic impact on the per-generation mutation rate—and the creation/ evolution debate.
Of the two published Y chromosome pedigree-based studies, both Xue et al. 2009 and Helgason et al. 2015 obtained Y chromosome mutation rates from low-coverage sequencing runs (i.e., 11× to 20× coverage). In contrast, whole genome sequencing of mtDNA in human pedigrees relies on high coverage sequencing runs [i.e., 40× (see S3 table in Ding et al. 2015) to >1000× (see main text of Guo et al. 2013, and main text of Rebolledo-Jaramillo et al. 2014)]. This contrast immediately suggests an explanation for the discrepancy in pedigree-based mutation rates for mtDNA versus the Y chromosome: discrepancies in the level of sequencing coverage. This hypothesis is testable.
However, to test this hypothesis, a second aspect of Y chromosome sequence quality must also be considered. Regardless of the level of coverage, raw or unfiltered sequencing reads from Y chromosome sequencing runs are not useful for pedigree-based mutation rate analyses. Compared to autosomes, the Y chromosome has an exceptional amount of sequences classes that make sequence read mapping especially challenging for reads derived from next-generation (i.e., short read) sequencing technology. Palindromic sequences, repetitive sequences, and sequences that easily map (i.e., with 99% identity) to the X chromosome lead to high levels of ambiguity in sequence read placement (Helgason et al. 2015; Poznik et al. 2013; Skaletsky et al. 2003). Thus, any study that attempts to analyze the results of Y chromosome sequencing runs must account for this mapping challenge.
Historically, several strategies have been employed to circumvent this mapping hurdle. For example, in the Xue et al. (2009) pedigree-based mutation rate study, the authors explicitly filtered out difficult-to-map regions of the Y chromosome. While they did not provide specific coordinates, they reported reducing the useful Y chromosome sequence from a euchromatic size of 24 megabases (Mb) to just 10.15 Mb (page 1454)—a reduction of over 50%. Conversely, in the Helgason et al. (2015) pedigree-based mutation rate study, the authors performed a type of filtration on candidate mutations. Rather than exclude mutations found in certain types of sequences classes, the authors weighed more heavily those mutations found in reliably mapping regions, and they decreased the weight of mutations found in ambiguously mapping regions.
With respect to other types of Y chromosome sequencing studies, such as large-scale mapping of the Y chromosome tree, similar filtering strategies have been employed. Some studies (Francalacci et al. 2013; Wei et al. 2013) have focused just on 8.97 Mb of the “X degenerate region” (XDG), a region known to be different enough from the X chromosome—and free enough of repetitive and palindromic sequences—to make straightforward the process of sequence read mapping. Other studies have empirically inferred regions of the Y chromosome that map uniquely, and these results tend to include the XDG plus scattered chunks from other sequence classes, resulting in a total of around 10.3 to 10.45 Mb of callable Y chromosome sequence (Poznik et al. 2013, 2016)— similar to the Xue et al. (2009) sequence size.
To date, two high coverage Y chromosome sequencing studies have been published in which pedigree-based mutation rate data are available (Karmin et al. 2015; Maretty et al. 2017). Both studies filtered their sequencing data via versions of previously published filters. Maretty et al. (2017) published a Y chromosome tree based on the sequence differences found on the XDG region of their male participants. Karmin et al. (2015) utilized a variety of filters, one of which (“filter c”) was explicitly based on the filters utilized by Wei et al. (2013) and Poznik et al. (2013). In this paper, we examine the pedigree-based mutation rates in high-coverage Y chromosome sequencing studies and explore the implications of these results for the Y chromosome molecular clock.
Materials and Methods
High coverage datasets
Karmin et al. (2015) dataset
From Karmin et al. (2015), we obtained the results of their high coverage sequencing runs on 24 Dutch father-son pairs, 6 Estonian father-son pairs, and 1 Estonian brother-brother pair from Table S2, column “FS” (i.e., Father-Son). We explored the Karmin et al. (2015) sequence filters that were reported in the text of the Supplementary Information.
Specifically, we focused on filter “c”, which was based on the previously published filters of Wei et al. (2013) and Poznik et al. (2013). Filter “c” reduced the mappable Y chromosome sequence to 10.79Mb. We also focused on the preferred filter of Karmin et al. (2015)—filter combination “a + b + d”. Filter “a” effectively eliminated regions where individuals had low coverage. Filter “b” effectively eliminated Y chromosome regions that also mapped to the X chromosome. Neither of these two filters in isolation reduced the callable region below 12 Mb. However, filter “d” alone reduced the callable region to 9.8Mb. This filter—the “re-mapping filter”—was one in which the authors “applied extrapolated information on next generation sequencing read mismapping areas, obtained from modelled Illumina datasets. These masks eliminate additional areas of low mappability on a short read level, inherent to genomic areas of frequent repeats as well as high sequence homology content” (page 7 of Supplemental Text). For this filter, the authors provided little to no measures of sensitivity and specificity. However, in combination with filter “a” and filter “b”, the filter “a + b + d” combination reduced the callable region to 8.8 Mb.
With respect to sequence coverage, the authors reported in the main text of the paper that “the Dutch father-son pairs were blood samples sequenced at >80× coverage” (page 463), but did not give specific information on the Estonian pairs. However, they stated that “sequencing of the whole genome was performed at Complete Genomics (Mountain View, California) at standard (>40×) coverage for blood- and high coverage (>80×) for saliva-based DNA samples” (page 463). We conservatively adopted 40× as the sequence coverage value for the Estonian samples.
Because of the pairing of the Y chromosome with the X chromosome, the Y chromosome exists in an effectively haploid state. Consequently, to calculate sequence coverage for the Y chromosome, we took the reported whole-genome sequencing coverage and divided it by 2.
To calculate the average Y chromosome sequence coverage for the entire Karmin study (i.e., Dutch + Estonian samples), we weighed each Y chromosome coverage value by the number of representatives present in the study. For example, for the Dutch:
For the Estonians:
Total weighing scheme:
30.97 + 4.52 = 35.5× average coverage
Maretty et al. (2017) dataset
While Maretty et al. (2017) reported a per-generation Y chromosome structural variant mutation rate, they did not explicitly report a per-generation Y chromosome single nucleotide mutation rate. However, Skov et al. (2017) utilized the Maretty et al. (2017) Y chromosome data; Skov et al. reported the existence in the Maretty et al. (2017) dataset of 62 males—including 17 father-son pairs—whose Y chromosome were assembled to high quality. Yet, like Maretty et al., Skov et al. also failed to report a per-generation Y chromosome single nucleotide mutation rate. Instead, Skov et al. (2017) relied on the low-coverage results of Helgason et al. (2015) to calibrate their data. Nevertheless, Maretty et al. (2017) published in Figure 4c of their paper a Y chromosome tree consisting of 62 males—likely the 62 males to which Skov et al. refer. In the Extended Data under the “Y-chromosome analysis” section, Maretty et al. detailed the construction of the tree: “The SNVs called using GATK above were used to construct the neighbour-joining tree. The SNVs were required to have a filter status of PASS, not be recurrent, and needed to be in the X-degenerate region. The neighbour-joining tree was constructed using MEGA6 (ref. 53) using the number of substitutions as the model and pairwise deletion as missing data treatment. It was run with 500 bootstrap replicates.”
We extracted the father-son SNV differences from the Figure 4c Y chromosome tree of Maretty et al. (2017) as follows: First, a screenshot was taken from the published pdf containing Figure 4c, and the screenshot included the associated scale bar of “50 mutations” (see Supplemental fig. 1). (The raw sequence data for the Y chromosomes is restricted access.) After electronically expanding the screenshot to a large size (while keeping the proportions constant), we located the 17 pairs with the shortest distances between them, to identify the 17 father-son pairs. Then we used the scale bar to visually and electronically estimate the nucleotide differences between each father-son pair (see Supplemental fig. 1 and Supplemental table 1).
These 17 nucleotide differences we then statistically tested for normality using the statistical software SPSS—both as raw values, and as results rounded to the nearest ones place. Both groups of data were a modest fit to a normal distribution based on visual inspection of histograms and Q-Q plots (Supplemental fig. 2) and based on statistical tests for normality (Kolmogorov-Smirnov and Shapiro- Wilk; see Supplemental table 2). Given these results, we calculated 95% confidence intervals using the t-distribution, and 95% confidence intervals based on bootstrapped analyses (see Supplemental tables 1 and 2), with 1,000 replications. We chose to use the t-distribution confidence interval since it was wider, and thus more conservative, than the bootstrapped confidence interval. Since mutations occur as whole units and not as decimals or fractions, we used the rounded values for further analyses.
We divided these numbers into the size of the sequence (“X-degenerate region”) analyzed—8.628 megabases (Mb)—to obtain a Y chromosome mutation rate in units of mutations per base pair per generation. Though Maretty et al. (2017) do not explicitly state the size of the X-degenerate region, they cite Helgason et al. (2015) when calibrating their mutation rates, and Helgason et al. specify a length of 8.6 Mb for the X-degenerate region. Also, Skov et al. (2017) imply in Figure 1a that the X-degenerate region is 8.628Mb in size (obtained by adding the values from the “total length” column for the 8 “X-deg” regions). We adopted the latter for our calculations.
The reported sequence coverage for Maretty et al. (2017) was 78× (page 87). Divided by 2, this would translate to a 39×. Skov et al. (2017) reported 40× coverage for the Y chromosomes in the dataset. We adopted the latter for our analyses.
Low coverage datasets
Xue et al. (2009) dataset
The reported sequencing coverage for each male in their two-person, 17-generation pedigree was 11× and 20× (page 1455). We averaged these values to 15.5×.
Helgason et al. (2015) dataset
The authors stated the average sequence coverage for the Y chromosomes to be 12.4× over the X-degenerate (XDG) regions.
For the identified mutations, their weights and the sequence classes to which they belonged were extracted from the Helgason et al. (2015) Table S4 and quantified in Excel (Supplemental table 3). The Y chromosome coordinates for these mutations were updated from NCBI36/hg18 values to GRCh38/hg38 values with the Lift Genome Annotations (LGA) tool (https://genome.ucsc.edu/cgi-bin/hgLiftOver). Since single positions do not update well, each individual mutation was assigned a coordinate pair that spanned 3 base pairs (the -1 and +1 positions surrounding the coordinate for the mutation, plus the mutation coordinate itself). These artificial coordinates were then updated with the LGA tool, and then the updated coordinate pairs (i.e., 3 base pair length) were converted back to single mutation coordinates (Supplemental table 4).
We tested the abilities of the Karmin et al. (2015) filters “c” and “a + b + d” (see above) to capture the various weights of these Helgason et al. (2015) mutations in several steps. First, we converted the coordinate positions for filters “c” and “a + b + d” to GRCh38/hg38 values with the LGA tool (see Supplemental tables 5–6 for conversion details, and for which regions were deleted/modified in the GRCh38/hg38 genome build). Second, the new coordinates for these filters were tested in Excel against the Helgason et al. (2015) mutations (with their respective GRCh38/hg38 coordinates) to see which Helgason et al. (2015) mutations were captured or rejected (see Supplemental tables 7–8 for details).
Mutation accumulation calculations
Mutation accumulation calculations (see Supplemental table 9) were performed by multiplying the derived high coverage (see above) Y chromosome single base pair mutation rate by the appropriate, model-specific time of origin. We did so by first converting these per-generation mutation rates to per-year rates, assuming a range of generation times (see Supplemental table 9). For the upper mutation rate value for our calculations, we used the upper bound of the t-distribution 95% confidence interval derived from Maretty et al. (2017) data. For the lower value for our calculations, we used the single value reported in the Karmin et al. (2015) dataset filter “c” column (they did not report a 95% confidence interval for this mutation rate).
We then applied the models-specific time of origin. Following Karmin et al. (2015), we adopted 250,000 years ago as the evolutionary time of origin for modern Homo sapiens. Conversely, for the YEC timescale, we adopted the post-Flood timescale. Because Genesis 9:18–19 indicates that all people alive today trace their ancestry back to the three sons of Noah, the Flood is a key time stamp for any genetic investigation. However, with respect to paternal inheritance of the Y chromosome, the Flood is the earliest time point accessible to us at present. Since Shem, Ham, and Japheth would have inherited their Y chromosomes from Noah, Noah’s Y chromosome is the earliest time point our studies could address, apart from pre-Flood fossil-derived Y chromosomes. Since no such fossil Y chromosome sequences are currently known, the earliest time point we can hope to model with our data is Noah’s birth.
For this date, we derived our temporal parameters from the details in Hardy and Carter (2014). Specifically, we adopted the Masoretic chronologies, but without taking a position on the debate on the length of the sojourn in Egypt. For our minimum age estimate, we used the Masoretic, Short Sojourn, Lunar Minimum dates; for the maximum age estimate, we use the Masoretic, Long Sojourn, Maximum dates supplied. We used these numbers to derive 2256 B.C. as the minimum date for the Flood, and 2646 B.C. as the maximum date for the Flood.
Technically, since Noah was born 600 years before the Flood (Genesis 7:11), we could have added 600 years to each of these dates, resulting in a minimum date of 2856 B.C. and a maximum date of 3246 B.C. However, in our calculations, we converted our per-generation mutation rate estimates to per-year estimates, using a range of average generation times. Noah’s age when we fathered his 3 sons (he was at least 500 years old; see Genesis 5:32) falls far outside this range. Hence, we simply adopted 2256 B.C. and 2646 B.C. as our minimum and maximum start dates, respectively, for the post-Flood timescale.
To fully round out our YEC time frame, we needed a “stop” date—the birth dates of the men in the Karmin et al. (2015) and Maretty et al. (2017) studies, from whom Y chromosome sequences were obtained. These dates were not given. However, we drew an analogy to the 1000 Genomes Project (“1KG”), since the 1KG-based Y chromosome paper (Poznik et al. 2016) was published around the same time as Karmin et al. (2015) and Maretty et al. (2017). The 1KG Project reported a minimum age for their participants as 18 years old,1 and indicated completion of the project by 2008.2 This implied a “stop” date of 1990 (i.e., A.D. 2008–18 years = A.D. 1990). Hence, we adopted A.D. 1990 as the maximum. To account for the fact that some men may have been young men while others may have been grandfathers, we also used A.D. 1950 as the minimum stop date (i.e., if we assume 1990 as the birth dates for the younger men, then 40 years earlier represents the 58 year old men—a rough surrogate value for the grandparental generation).
We compared these mutation accumulation predictions to the branch lengths derived from the data in Karmin et al. (2015). Since the published tree in Karmin et al. was given in time units rather than in units of base pairs, we redrew a tree from the Karmin et al. data. First, we converted the online supplied VCF file3 to FASTA format with PGDSpider software,4 after applying appropriate sample names and labels to each individual based on the online metadata (see Supplemental Table 10 for details of relabeling).5 Though the tree in the main text of Karmin et al. contains over 300 individuals, the metadata file contains labels for only 297. Then we drew a midpoint-rooted neighbor-joining tree (see Supplemental fig. 3 for rectangular display, Supplemental fig. 4 for topology-only display) with MEGA7 (Kumar, Stecher, and Tamura 2016) software, selecting the “Pairwise deletion” option for treatment of gaps/missing data. Branch lengths were extracted manually from the tree and deposited in Supplemental table 11).
We calculated the average and standard deviation for the branch lengths based on two different root positions. The first root (“Evo”) used the leftmost (Supplemental fig. 3–4; Supplemental table 11) split position as the root of the tree. As per the accompanying Jeanson (2019) paper, we also tested branch lengths based on the “Alpha” root—which we defined in Supplemental figs. 3–4 and in Supplemental Table 11 as the point halfway (i.e., 62 base pairs, or half of 124 base pairs) between nodes 483 and 511.
Results
High coverage sequencing increases pedigree-based mutation rate
From the Karmin et al. 2015 and Maretty et al. 2017 datasets, we extracted the per-generation pedigree-based mutation rates using standard filtering criteria. For the Karmin et al. study, several filter options were supplied, and we chose filter “c” since it corresponded to previously published criteria. Consistent with what is already known from comparisons of low coverage and high coverage sequencing runs (Poznik et al. 2016), the two high coverage datasets revealed a per-generation mutation rate that was, on average, 10- to 17-times faster (table 1) than the previously published (i.e., Xue et al. 2009; Helgason et al. 2015) low coverage studies. This suggested that the real per-generation Y chromosome single nucleotide mutation rate was much higher than previously determined. In fact, it suggested that, in the future, sequence runs at even higher coverage values might further increase this value.
Xu et al. 2009 | Helgason et al. 2015 | Karmin et al. 2015 | Maretty et al. 2017 | |
---|---|---|---|---|
Sequence coverage (given in units of fold-coverage | 15.5 | 12.4 | 35.5 | 40 |
Average mutation sper base pair per generation | 3.0E-08 | 3.00E-08 | 3.02E-07 | 5.0E-07 |
95% confidence interval | 8.9E-9 to 7.0E-8 | 2.85E-8 to 3.16E-8 | (not given) | 3.5E-07 to 6.4E-07 |
Failure of counter-explanations
Counter-explanations for these results came from only one of these two studies. With respect to Maretty et al. (2017) [and the corresponding study in Skov et al. (2017)], the authors appeared to possess the raw data indicating a high per-generation Y chromosome mutation rate. However, no comment on these rates were made.
In contrast, Karmin et al. (2015) attempted to explain the unusually high mutation rate that they discovered by employing additional filtration steps to the Y chromosome sequence reads. However, rather than strengthen their counter-explanations, their attempts strengthened the original implications of their findings.
Two lines of evidence supported this contention. First, Karmin et al. (2015) employed a logically circular argument to explain away the high mutation rate. In the Supplemental Text, they explained that their rationale for explaining away the high rate was not a new discovery about the ambiguity of sequence read mapping. Rather, they stated that “we initially applied a combination of regional filters previously defined on the basis of analyses of Illumina HiSeq data (Poznik et al. 2013; Wei et al. 2013a), resulting in ten regions of Chr Y sequence, altogether capturing 10.8 Mb (filter c, Table S2). However, the application of the regional filters led only to a modest reduction of false positive calls judged by the number of father-son/brother-brother (FS) differences and the count of recurrent mutations (Table S2)” (page 4). (A higher mutation rate would also lead to more recurrent mutations; thus, attempts to reduce father-son/brother-brother mutation rates and the number of recurrent mutations are, essentially, two forms of achieving the same goal.) In other words, the Karmin et al. test for false positives was an evolutionarily-defined low mutation rate.
This was made even more clear in how Karmin et al. (2015) defined the accuracy of their filtering strategy: “The number of FS [i.e., Father-Son] differences was approximately 10-fold higher than the expected number of de novo mutations considering the range of published Chr Y mutation rates (Xue et al. 2009; Francalacci et al. 2013; Mendez et al. 2013; Poznik et al. 2013). This finding prompted us to explore additional filters” (page 4 of the Supplementary Information). Of the four studies they cited—Xue et al. 2009; Francalacci et al. 2013; Mendez et al. 2013; Poznik et al. 2013—only the Xue et al. study represented a pedigree-based Y chromosome mutation rate. The other three studies derived a mutation rate via the historically circular evolutionary geology-based molecular clock method—see Introduction—or by extrapolating the autosomal mutation rate onto the Y chromosome.
Second, independent tests of the specificity and sensitivity of the Karmin et al. (2015) extra filtration steps revealed a slight gain in specificity at the expense of a large loss in sensitivity. Unfortunately, in the Karmin et al. (2015) description of their uniquely defined 8.8 Mb filter “a + b + d”—the filter combination that achieved a lower Y chromosome mutation rate—the authors reported no results on the specificity and sensitivity of their filtration strategy. Aside from their circular attempts to reduce the father-son mutation rate to a value in line with what their evolutionary expectations defined, the authors provided no checks and balances on their methods.
However, shortly after the Karmin et al. (2015) paper appeared in print, Helgason et al. (2015) reported their pedigree-based mutation rate (based on low coverage sequencing runs) that happened to fall in line with evolutionary expectations, and also fell in line with the previously published low coverage results from Xue et al. (2009). In principle, we might expect the authors of Karmin et al. (2015) to endorse the Helgason et al. (2015) results and conclusions. Thus, we can use the Helgason et al. (2015) results to test the Karmin et al. (2015) filters for sensitivity and specificity.
Since Helgason et al. weighed their mutation results based on mapping—rather than based on some evolutionary-defined endpoint—we can evaluate the Karmin et al. filters based on their ability to reproduce the Helgason et al. results. To test for specificity, we can examine which Karmin et al. filters call the least number mutations to which Helgason et al. assigned a low weight. To test for sensitivity, we can examine which Karmin et al. filters capture the most mutations to which Helgason et al. assigned a full weight.
We found that both filter “c” and filter “a + b + d” from Karmin et al. (2015) rejected—filtered out—the vast majority of the low weight Helgason et al. (2015) mutations (table 2). As expected, the more stringent filter “a + b + d” rejected more mutations than filter “c.” However, neither filter captured all of the high weight Helgason et al. (2015) mutations (table 2). Nevertheless, filter “c” retained many more high weight mutations than filter “a + b + d.”
Filter | Unweighted (reliable) mutations | Weighted (unreliable) mutations |
---|---|---|
(None–original Helgason et al. 2015 data) | 1015 | 1035 |
Karmin et al. 2015 filter c | 718 | 15 |
Karmin et al. 2015 filter abd | 593 | 3 |
Quantifying these results in terms of percentages, we found that both filters rejected >98% of the low weight mutations—with the difference between filter “a + b + d” and filter “c” being just 1.2% of the low weight mutations (table 3). Conversely, filter “c” retained 70.7% of the high weight Helgason et al. mutations, whereas filter “a + b + d” retained only 58.4%—a loss of 12.3% of the high weight mutations. Thus, by the independent test of the filtering strategy of Helgason et al. (2015), the Karmin et al. filter “a + b + d” is more stringent that the more commonly used filter “c”, yet the gain in specificity is offset by the large loss in sensitivity. This fact demonstrated that the Karmin et al. filter “a + b + d” was excessively stringent and that it artificially reduced the mutation rate to a value less than it is.
Filter | Sensitivity (% of reliable Iceland data retained | Specificity (% of unreliable Iceland retained |
---|---|---|
Karmin et al. 2015 filter c | 70.7 | 1.4 |
Karmin et al. 2015 filter abd | 58.4 | 0.3 |
Loss/Gain | -12.3 | 1.2 |
Subsequent Y chromosome sequencing efforts from other investigators did not employ the Karmin et al. (2015) filter “a + b + d.” For example, to date, one of the largest global analyses of human paternal genetic history is the 1000 Genomes Project. From the 1,244 Y chromosome sequences in this project, a tree was constructed using the mapping-based 10.3Mb filter (Poznik et al. 2016)—not the 8.8 Mb filter “a + b + d.”
Together, these results strengthened the original implications (i.e., a higher mutation rate than previous studies had found) of Karmin et al.’s high coverage sequencing results.
High coverage mutation rate explains Y chromosome differences in 4,500 years
We found that the mutation rates from the high coverage studies explained the branch lengths of the Y chromosome tree within just a few thousand years (fig. 1). We also found that these rates rejected the evolutionary time of origin for the first modern Homo sapiens (fig. 2). For simplicity, when measuring total branch lengths, we began by simply adopting the typical evolutionary root position. Conversely, based on the results of the accompanying paper (Jeanson 2019), we also explored an alternative, better-supported (see Jeanson 2019) root position, and we found that the high coverage Y chromosome mutation rate explained all but the most divergent haplogroup A branch lengths in about 4,500 years (fig. 3).
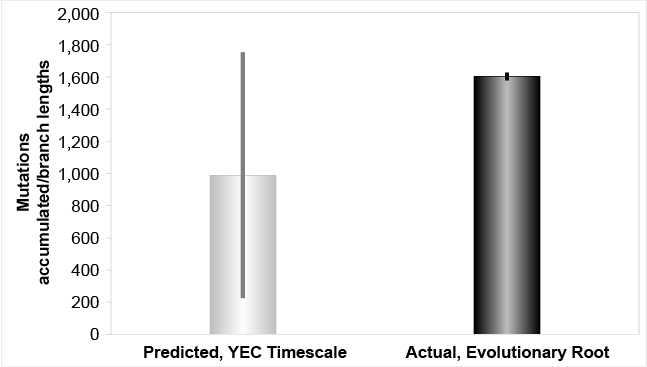
Fig. 1. Mutation accumulation in the Y chromosome over the young-earth creation (YEC) timescale, evolutionary root. The derived Y chromosome pedigree-based mutation rates from high coverage sequencing runs were converted to units of mutations per year and multiplied over the YEC timescale. These predictions were compared to the branch lengths derived from Karmin et al. (2015) data, based on the typical evolutionary root position, and these predictions captured the branch length values.
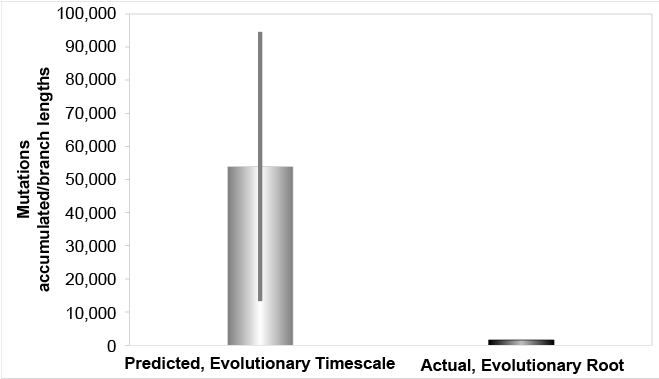
Fig. 2. Mutation accumulation in the Y chromosome over the evolutionary timescale, evolutionary root. The derived Y chromosome pedigree-based mutation rates from high coverage sequencing runs were converted to units of mutations per year and multiplied over the evolutionary timescale. These predictions were compared to the branch lengths derived from Karmin et al. (2015) data, based on the typical evolutionary root position. The evolutionary predictions over-predicted the branch length values by 8- to 59-fold.
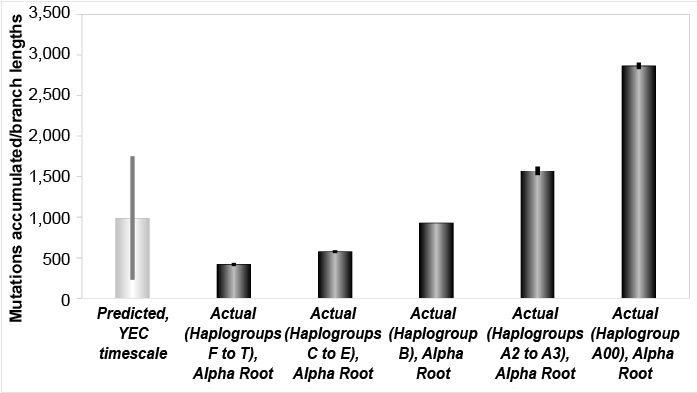
Fig. 3. Mutation accumulation in the Y chromosome over the young-earth creation (YEC) timescale, alternate root. The derived Y chromosome pedigree-based mutation rates from high coverage sequencing runs were converted to units of mutations per year and multiplied over the YEC timescale. These predictions were compared to the branch lengths derived from Karmin et al. (2015) data, based on the Alpha (Jeanson 2019) root position, and these predictions captured all but the A00 branch length values.
These latter results predicted a higher per-generation mutation rate for the most divergent A00 individuals. In addition, because this same root position shows a gradient of branch lengths (fig. 3), Fig. 3 implied a gradient of per-generation mutation rates, depending on exactly which root position (i.e., Gamma, Epislon, or Alpha—or somewhere in between; see Jeanson 2019) turned out to be correct.
Finally, these results made indirect predictions about the relationship between the history of civilization and the structure of the Y chromosome tree. Since phylogenetic trees record changes in population size (e.g., see Karmin et al. 2015), the current Y chromosome tree must have also recorded changes in past human population size. However, since our results implied that the entire tree was only a few thousand years old, our results predicted that known recent (i.e., with in the last few thousand years) changes in population size would be stamped throughout the tree in a manner consistent with the recent origin of the tree. In other words, the deeper roots of the Y chromosome tree should record not changes in population size from 200,000 years ago but changes in population size from the recent past (see accompanying Jeanson 2019 paper).
Discussion
A 4,500-year-old Y chromosome molecular clock
Our results demonstrate that a Y chromosome molecular clock exists, and that it specifies about 4,500 years in total for human paternal history (figs. 1, 3). Rather than being an anomaly, these results fall in line with the expectations derived from comparisons of low coverage and high coverage Y chromosome sequences (Poznik et al. 2016). Since high coverage sequencing is known to increase the tree tip length over the lengths derived from low coverage sequencing, and since father-son relationships among the living represent the most terminal aspect of any tree, our empirical results match precisely what previous results had predicted.
Conversely, our results also strongly challenge the evolutionary timescale (fig. 2). Rather than confirm a history for humanity that stretches back hundreds of thousands of years, these results reject this hypothesis. If men have been around for hundreds of thousands of years, they should have accumulated mutations 8- to 59-times the amount currently observed. Instead, we observe only a few thousand years’ worth of mutation accumulation.
Furthermore, the combined results from mtDNA (i.e., Jeanson 2013, 2015, 2016) and Y chromosome (i.e., this paper) analyses represent two independent lines of evidence—maternal ancestry and paternal ancestry—that reject the evolutionary timescale for the origin of humans. Together, these two datasets falsify the current evolutionary model for humanity.
How might the evolutionary model adapt to these contrary data? With respect to pedigree-based analyses, evolutionists might invoke natural selection—a mechanism by which a high mutation rate could be converted to a lower substitution rate. Alternatively, evolutionists might hypothesize that the mutation rate has recently sped up—that it was much slower in times past. Either way, for these hypotheses to be scientific they must make testable predictions. For example, can any of these evolutionary hypotheses predict the pedigree-based mutation rate for A00 individuals? Or African individuals in general (since no African father-son pedigrees were part of the Karmin et al. 2015 and Maretty et al. 2017 studies)? Or Asian individuals, or Native American individuals (none of whom were part of these same father-son pedigree studies)? Unless the evolutionary hypotheses can meet this standard (i.e., the standard to which evolutionists have held creationists for many years; see Eldredge 1982 and Futuyma 2013), then these hypotheses cannot be considered scientific.
In contrast, our YEC-confirming results led to several testable predictions. These testable predictions add scientific weight to the YEC conclusions found in this paper.
Other Ways to Rescue the Evolutionary Model?
One finding reported in Karmin et al. (2015) could give pause to the conclusions reached in this paper. In the Supplemental Text, Karmin et al. report that they “validated with Sanger sequencing SNPs between three fathers and their six sons and between two brothers in one case where father’s genome failed QC. We compared altogether seven pairs from four Estonian families. At first we applied VQHIGH, 95% call-rate, custom filter combination (a + b + d) and excluded all individual N-s from comparisons. This filtering scheme revealed within these seven pairs in total 6 differences. All these occurred within two families and in 4 unique positions. These were 1) two positions where both fathers had each one position where they were in derived state but their two sons in reference state, thus implicating a possible sequencing error (or back mutation); 2) two unique positions where one father had two sons carrying one derived allele each, implicating possible mutation between generations. To see the effect of different filtering we also used only filters b + d which revealed that both sons from the family already carrying four differences have each one additional derived allele. For these six SNV-s we were able to design Chr Y specific primers and to get homozygous Chr Y sequence calls for five of these positions. Sanger sequencing revealed that all of the studied positions were false positives” (page 7). In other words, these false positives might imply that the high mutation rate under filter “c” was an artifact, not a real finding.
However, in this particular example, the authors once again failed to address questions of sensitivity and specificity. In this case, let’s indirectly examine these metrics by exploring other statements that the authors made about their own work. For example, they stated in the main text that “Data quality assessment by evaluating SNP differences between father-son pairs resulted in an average of approximately one mutation per pair, indicating a low false-positive rate” (page 461). This conclusion refers to Table S2 of their paper, which shows a father-son mutation rate of 0.62 mutations per generation. Yet their Sanger sequencing results implied that, in the father-son pairs that they examined, the mutation rate was less than 1 mutation every 7 generations, not 1 every 1.6 generations (i.e., 0.62 mutations per generation). The authors do not seem to perform consistent or comprehensive quality control on their samples.
Perhaps this is because the results would have been too stringent even for the ancient evolutionary timescale. Extrapolated to the rest of the father-son pairs, the Sanger sequencing results imply that all father-son differences were false positives, which would produce a mutation rate less than 1 mutation every 31 generations—10 times lower than expectations based on a model which puts the first male ancestor about 250,000 years ago.
The authors’ “false positive” findings raise even more questions about the reliability of their entire tree. In other words, given the authors’ stated motivation for reducing the father-son rate—namely, bringing the results into alignment with evolutionary expectations, what happens to their results when we apply their quality control logic across the entire tree (i.e., across the tree albeit free of circular evolutionary reasoning)? If the only father-son variants that they tested by rigorous quality control methods were false positives, how do we know that any variants in the tree were real? That is, aside from invoking evolution as the answer, what consistent, comprehensive quality control measures did the authors employ to give us confidence that their entire tree was reliable?
In short, the Karmin et al. (2015) attempts to identify positive controls via Sanger sequencing add little of scientific value to our discussion. They raise more questions than they answer, and they cannot be explored further until these larger questions are addressed.
Conversely, one other reason for pause stems from the methodologies we employed with the Maretty et al. (2017) data. Since our mutation rate was derived from inferring branch lengths from their published tree, it will be important to confirm these results with the raw (i.e., pairwise alignment-based rather than phylogenetic tree-based) Y chromosome sequences from the father-son pairs. We anticipate little deviation from the results we described here.
Conclusion
Together, the results in this paper and in the accompanying paper (Jeanson 2019) present a compelling case for the origin of the most recent, globally common human male ancestor within the last 4,500 years. Combined with the previously published results from mtDNA (Jeanson 2016), our data also make the YEC origin of the most recent common male and female ancestors a difficult conclusion to refute.
Acknowledgments
Discussions with Rob Carter have been invaluable in tackling the question of the Y chromosome molecular clock. His generous sharing of unpublished results has paved the way for the current study. Reviewers of this work also helped refine and sharpen the manuscript. One reviewer in particular supplied very helpful suggestions and methodological tips.
References
Carter, Robert W., Stephen S. Lee, and John C. Sanford. 2018. “An Overview Of the Independent Histories Of the Human Y Chromosome and the Human Mitochondrial Chromosome.” In Proceedings of the Eighth International Conference on Creationism. Edited by J. H. Whitmore, 133– 151. Pittsburgh, Pennsylvania: Creation Science Fellowship.
Coventry, A., L. M. Bull-Otterson, X. Liu, A. G. Clark, T. J. Maxwell, J. Crosby, J. E. Hixson, et al. 2010. “Deep Resequencing Reveals Excess Rare Recent Variants Consistent With Explosive Population Growth.” Nature Communications 1 (November 30): 131.
Ding, Jung, Carlo Sidore, Thomas J. Butler, Mary Kate Wing, Yong Qian, Osorio Meirelles, Fabio Busonero, et al. 2015. “Assessing Mitochondrial DNA Variation and Copy Number in Lymphocytes of ~2,000 Sardinians Using Tailored Sequencing Analysis Tools.” PLoS Genetics 11, no. 7 (July 14): e1005306.
Eldredge, Niles. 1982. The Monkey Business: A Scientist Looks at Creationism. New York: Washington Square Press.
Francalacci, Paolo, Laura Morelli, Andrea Angius, Riccardo Berutti, Frederic Reinier, Rossano Atzeni, Rosella Pilu, et al. 2013. “Low-Pass DNA Sequencing Of 1200 Sardinians Reconstructs European Y-Chromosome Phylogeny.” Science 341, no. 6145 (2 August): 565–569.
Frello, Stefan. 2017a. “On the Creationist View on mtDNA.” Answers Research Journal 10 (August 23): 181–182. https://answersingenesis.org/human-evolution/creationist-view-mtdna/.
Frello, Stefan. 2017b. “Reply to ‘Response to “On the Creationist View on mtDNA”’.” Answers Research Journal 10 (October 4): 237. https://answersingenesis.org/human-evolution/reply-to-response-to-creationist-view-mtdna/.
Futuyma, Douglas J. 2013. Evolution. Sunderland, Massachusetts: Sinauer Associates, Inc.
Fu, Wenqing, Timothy D. O’Connor, Goo Jun, Hyun Min Kang, Goncalo Abecasis, Suzanne M. Leal, Stacey Gabriel, et al. 2013. “Analysis of 6,515 Exomes Reveals the Recent Origin of Most Human Protein-Coding Variants.” Nature 493, no. 7431 (10 January): 216–220.
Guo, Yan, Chung-I Li, Quanhu Sheng, Jeanette Falck Winther, Qiuyin Cai, John Boice, and Yu Shyr. 2013. “Very Low-Level Heteroplasmy mtDNA Variations Are Inherited in Humans.” Journal of Genetics and Genomics 40, no. 12 (December): 607–615.
Hardy, Chris, and Robert Carter. 2014. “The Biblical Minimum and Maximum Age of the Earth.” Journal of Creation 28, no. 2 (August): 89–96.
Helgason, Agnar, Axel W. Einarsson, Valdís B. Guõmundsdóttir, Ásgeir Sigurõsson, Ellen D. Gunnarsdóttir, Anuradha Jagadeesan, S. Sunna Ebenesersdóttir, et al. 2015. “The Y-Chromosome Point Mutation Rate In Humans.” Nature Genetics 47, no. 5 (25 March): 453–457.
Jeanson, Nathaniel T. 2013. “Recent, Functionally Diverse Origin For Mitochondrial Genes From ~2700 Metazoan Species.” Answers Research Journal 6 (December 11): 467– 501. https://answersingenesis.org/genetics/mitochondrial-dna/recent-functionally-diverse-origin-for-mitochondrial-genes-from-~2700-metazoan-species/.
Jeanson, Nathaniel T. 2015. “A Young-Earth Creation Human Mitochondrial DNA “Clock”: Whole Mitochondrial Genome Mutation Rate Confirms D-loop Results.” Answers Research Journal 8 (September 23): 375–378. https://answersingenesis.org/genetics/mitochondrial-genome-mutation-rate/.
Jeanson, Nathaniel T. 2016. “On the Origin of Human Mitochondrial DNA Differences, New Generation Time Data Both Suggest a Unified Young-Earth Creation Model and Challenge the Evolutionary Out-of-Africa Model.” Answers Research Journal 9 (April 27): 123–130. https://answersingenesis.org/genetics/mitochondrial-dna/origin-human-mitochondrial-dna-differences-new-generation-time-data-both-suggest-unified-young-earth/.
Jeanson, Nathaniel T. 2017a. “Response to ‘On the Creationist View on mtDNA’.” Answers Research Journal 10 (August 23): 183–186. https://answersingenesis.org/human-evolution/response-creationist-view-mtdna/.
Jeanson, Nathaniel T. 2017b. “Response to ‘Reply to “Response to ‘On the Creationist View on mtDNA’”’.” Answers Research Journal 10 (October 4): 239–240. https://answersingenesis.org/human-evolution/response-to-reply-to-response-to-creationist-view-mtdna/.
Jeanson, Nathaniel T. 2019. “Testing the Predictions of the Young-Earth Y Chromosome Molecular Clock: Population Growth Curves Confirm the Recent Origin of Human Y Chromosome Differences.” Answers Research Journal 12: 405–423.
Jeanson, Nathaniel T. and Jason Lisle. 2016. “On the Origin of Eukaryotic Species’ Genotypic and Phenotypic Diversity: Genetic Clocks, Population Growth Curves, and Comparative Nuclear Genome Analyses Suggest Created Heterozygosity in Combination with Natural Processes as a Major Mechanism.” Answers Research Journal 9 (April 20): 81–122. https://answersingenesis.org/natural-selection/speciation/on-the-origin-of-eukaryotic-species-genotypic-and-phenotypic-diversity/.
Karmin, Monica, Lauri Saag, Mário Vicente, Melissa A. Wilson Sayres, Mari Järve, Ulvi Gerst Talas, Siiri Rootsi, et al. 2015. “A Recent Bottleneck of Y Chromosome Diversity Coincides with a Global Change in Culture.” Genome Research 25, no. 4 (April): 459–466.
Keinan, Alon, and Andrew G. Clark. 2012. “Recent Explosive Human Population Growth Has Resulted in an Excess of Rare Genetic Variants.” Science 336, no. 6082 (May): 740–743.
Kumar, Sudhir, Glen Stecher, and Koichiro Tamura. 2016. “MEGA7: Molecular Evolutionary Genetics Analysis Version 7.0 For Bigger Datasets.” Molecular Biology and Evolution 33, no. 7 (July): 1870–1874.
Maretty, Lasse, Jacob Malte Jensen, Bent Petersen, Jonas Andreas Sibbesen, Siyang Liu, Palle Villesen, Laurits Skov, et al. 2017. “Sequencing and De Novo Assembly of 150 Genomes from Denmark as a Population Reference.” Nature 548 (3 August): 87–91.
Nelson, Matthew R., Daniel Wegmann, Margaret G. Ehm, Darren Kessner, Pamela St. Jean, Claudio Verzilli, Judong Shen, et al. 2012. “An Abundance of Rare Functional Variants in 202 Drug Target Genes Sequenced in 14,002 People.” Science 337, no. 6090 (6 July): 100–104.
Poznik, G. David, Brenna M. Henn, Muh-Ching Yee, Elzieta Sliwerska, Ghia M. Euskirchen, Alice A. Lin, Michael Snyder, et al. 2013. “Sequencing Y Chromosomes Resolves Discrepancy in Time to Common Ancestor of Males Versus Females.” Science 341, no. 6145 (2 August): 562–565.
Poznik, G. David, Yali Xue, Fernando L. Mendez, Thomas F. Willems, Andrea Massaia, Melissa A. Wilson Sayre, Qasim Ayub, et al. 2016. “Punctuated Bursts in Human Male Demography Inferred from 1,244 Worldwide Y-Chromosome Sequences.” Nature Genetics 48, no. 6 (25 April): 593–599.
Rebolledo-Jaramillo, Boris, Marcia Shu-Wei, Nicholas Stoler, Jennifer A. McElhoe, Benjamin Dickins, Daniel Blankenberg, Thorfinn S. Korneliussen, et al. 2014. “Maternal Age Effect and Severe Germ-Line Bottleneck in the Inheritance of Human Mitochondrial DNA.” Proceedings of the National Academy of Sciences USA 111, no. 43 (October 13): 15474–15479.
Sanford, John C., Robert W. Carter, Wes Brewer, John Baumgardner, and Bruce Potter. 2018. “Adam and Eve, Designed Diversity, and Allele Frequencies.” In Proceedings of the Eighth International Conference on Creationism. Edited by J. H. Whitmore, 200–216. Pittsburgh, Pennsylvania: Creation Science Fellowship.
Skaletsky, Helen, Tomoko Kuroda-Kawaguchi, Patrick J. Mix, Holland S. Cordum, LeDeana Hillier, Laura G. Brown, Sjoerd Repping, et al. 2003. “The Male-Specific Region of the Human Y Chromosome is a Mosaic of Discrete Sequence Classes.” Nature 423, no. 6942 (19 June): 825–837.
Skov, Laurits, The Danish Pan Genome Consortium, and Mikkel Heide Schierup. 2017. “Analysis of 62 Hybrid Assembled Human Y Chromosomes Exposes Rapid Structural Changes and High Rates of Gene Conversion.” PLoS Genetics 13, no. 8 (August 28): e1006834. https://doi.org/10.1371/journal.pgen.1006834.
Tennessen, Jacob A., Abigail W. Bighma, Timothy D. O’Connor, Wenqing Fu, Eimear E. Kenny, Simon Gravel, Sean McGee, et al. 2012. “Evolution and Functional Impact of Rare Coding Variation From Deep Sequencing of Human Exomes.” Science 337, no. 6090 (6 July): 64–69.
Wei, Wei, Qasim Ayub, Yuan Chen, Shane McCarthy, Yipping Hou, Ignazio Carbone, Yali Xue, and Chris Tyler-Smith. 2013. “A Calibrated Human Y-Chromosomal Phylogeny Based On Resequencing.” Genome Research 23, no. 2 (October 4): 388–395.
Xue, Yali, Qiuju Wang, Quan Long, Bee Ling Ng, Harold Swerdlow, John Burton, Carl Skuce, et al. 2009. “Human Y Chromosome Base-Substitution Mutation Rate Measured By Direct Sequencing In a Deep-Rooting Pedigree.” Current Biology 19, no. 17 (September 15): 1453–1457.